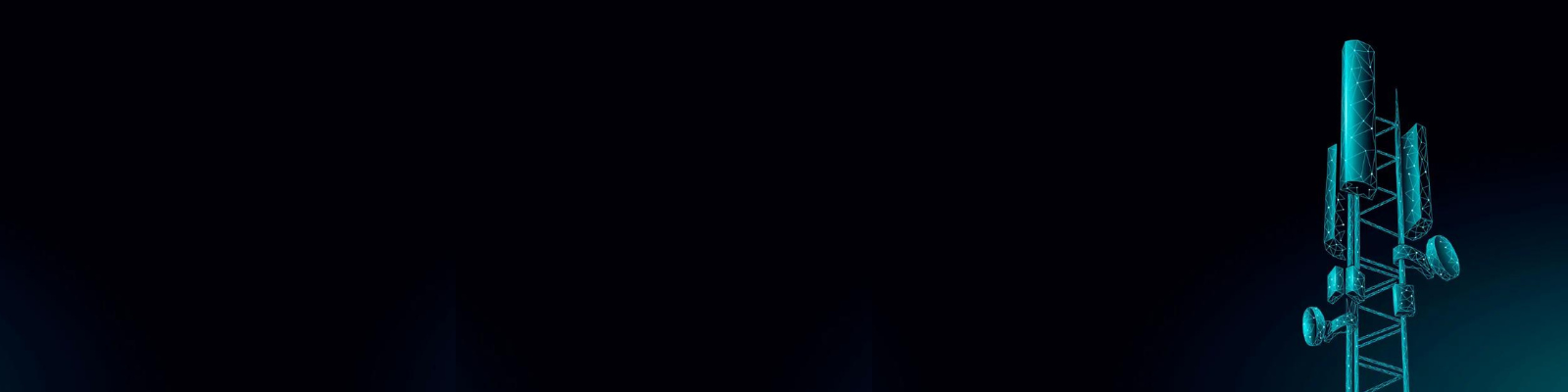
BLOG POST
Why do CSPs need a little help from their cloud-scaler friends?
Cloud-based options for data processing at massive scale offer attractive options – but you need the right toolkit to cater for rich telco data – and the right approach that meets your goals for security and privacy. Partnership makes sense.
The relationship between CSPs and cloud-scalers is subject to numerous discussions. But, moving aside from chatter regarding network investments and data flows, one thing’s abundantly clear: CSPs and cloud-scalers need each other.
There are many reasons why, but in this blog, we’ll focus on just one: data processing, at scale. Of course, what we’re talking about here is telco data – network events, signaling captured by probes, location information, customer CDRs, device details and so on – plus, all the user data customers generate.
Can data processing keep pace with data growth?
We all know that these data volumes are exploding – and a quick look at the latest Mobility Report from Ericsson provides all the additional confirmation we need. According to this long-running and widely respected research project, global mobile data traffic is expected to reach 325 EB per month in 2028 – a roughly fourfold increase when compared to 2022. As might be expected, this is also reflected in predicted data growth from individual smartphones. Ericsson reckons this will grow from 19GB per device in 2023 to 46 GB by the end of 2028.
That’s a lot of data – and we’re not even considering that from fixed networks, or fixed wireless access connectivity – or the IoT, come to that. So, whichever way we cut it, there’s a lot of data. Now, the real problem here is that the industry is united in its conviction that this data contains a dazzling array of insights that will help us to deliver better services and richer experiences for customers – while also enabling us to run our networks more efficiently.
To cope with the large amounts of data, we want to shift to machine-learning based DataOps processes and frameworks, so that we can ingest data from any source and then process it in a consistent and accessible manner – which we explored in a recent post.
But this raises an obvious question: where do we put this infrastructure? And another: how do we ensure that it can scale to keep pace with growth? After all, if we expect the data volume to grow by as many as four times in just five years, we need to ensure that investments today can stretch to satisfy demand.
Do we use the cloud, on-prem or hybrid?
And that’s why we need help from the hyper-scalers. Yes, you could build an entire data mesh infrastructure on your own premises, but that’s a huge task. In truth, it’s a task that few can even contemplate. In contrast, hyper-scalers offer an existing, software-based computing infrastructure that can scale without limits – and has been built from the ground up as cloud native.
So, what we need is the ability to leverage this hyper-scale infrastructure, by taking cloud-agnostic platforms that can consume data (from any source), analyze data and deliver value – and putting them into the cloud where machine learning-based DataOps can run effectively.
However, that’s not to say that this is an entirely binary choice. We definitely see that hybrid options can also be leveraged – it’s just that to get the true scale we need, we must use the elastic data processing capabilities for which the cloud was designed. We can’t simply replicate or rebuild this infrastructure in its entirety; that would be costly, time-consuming – and likely be overwhelmed as data volumes continue to surge. In addition, a hybrid infrastructure can also help CSPs manage regulatory requirements by enabling restricted or protected data to be kept on premise, while other data is shifted to the cloud.
Tools to help you navigate the right course
The point is that we all have great ambitions for processing and deriving insights from network, service, and customer data. We can absolutely extract these insights – to drive optimization, enhance performance and support automated operations, among other applications. We have the tools to help you do this and they are proven, offering the specific parsing capabilities and data capture techniques we need for handling unique telco data.
But, to ensure we can continue on this pathway while data volumes surge, and as we integrate new sources of data, we need partners from the hyper-scale community to do it.
Elisa Polystar can help you get there, with an open architecture that can reside on both cloud and hybrid cloud infrastructure, enabling you to process your data at scale, based on best-of-breed components and without creating long term lock-in.